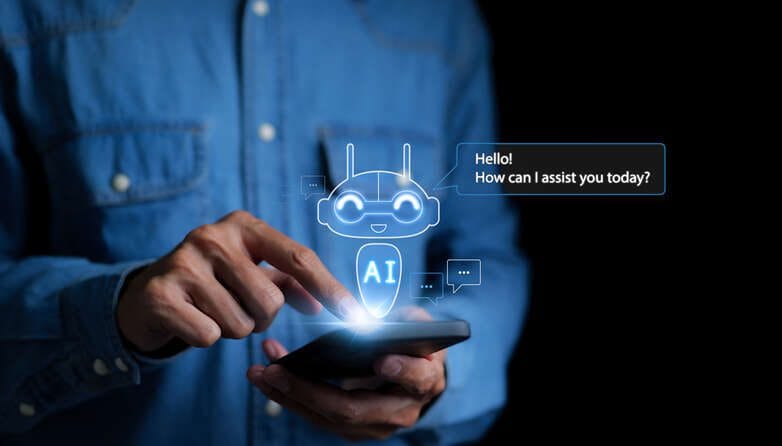
The evolution of AI in last-mile technology
By Bob Griffiths, Chief Strategy Officer, Doddle
Last mile or final mile deliveries are some of the most resource-intensive and expensive parts of the delivery journey and with the ecommerce boom showing no signs of slowing down the world’s largest companies are embracing technologies such as Artificial Intelligence (AI) to help cut costs at this integral stage in the journey.
Intelligent delivery and returns
Today, businesses like Amazon and retailers using Doddle’s Digital Returns platform use rules-based intelligence to deal with returns based on a list of retailer-defined options. These rules are created by the retailer to balance operational efficiency and customer loyalty.
The best digital returns solutions can be customised based on pre-configured rules, (basic, user-created algorithms) which intelligently adapt the options and costs presented to consumers based on order information, item details, dimensions, category or previous returns behaviour. The processing is then configured to treat items differently depending on data.
Amazon is the market leader in this type of ‘rules engine’, (from both the retail and carrier perspectives) allow customers to initiate a return from their order list, where their available options (and pricing of those options) are determined by various factors, including their return reason, the type of item being returned, the cost to Amazon of the return, and the availability of local Amazon-owned drop-off points. This means that damaged items or late deliveries are usually eligible for free return shipping and refund. ‘Buyer’s remorse’ returns are usually charged, the cost of which varies depending on the drop-off method.
Amazon has used these return charges in the past to incentivise adoption and utilisation of its locker network, its pickup counter Hub network, and other first-party return locations such as Whole Foods grocery stores.
However, for most carriers who offer a digital returns solution, pricing a return according to the reason for that return is very rare. Rules-based intelligence is not commonly available, and where it is, the list of available inputs and outputs is limited.
It’s even rarer for the customer insights generated in an intelligent returns process to be integrated with other tools or systems such as a CRM platform, meaning the carrier/retailer won’t be able to use the insights collected from the huge quantity of data that such a returns solution could capture.
While rules engines can theoretically allow a significant degree of personalisation, it’s very difficult to decide which variables to include and even more difficult to integrate these inputs into the engine. That’s true within businesses (integrating between systems and departments) and between businesses (for example, a parcel carrier integrating with a retailer to utilise customer data to offer a personalised set of delivery or returns options).
Even where machine learning and artificial intelligence does exist in logistics tech, it’s often isolated from other useful data. Neural networks or routing algorithms have been used to determine efficient parcel routing for years but they don’t consider carding or what time of day deliveries are most likely to succeed, which could make them even more efficient.
AI in chatbots
Chatbots are an obvious way for AI to support ecommerce logistics. However, they’re not always welcomed by all customers, many of whom would much rather talk to a customer service representative. However, chatbots can have a role outside of customer support.
XPO, a European 3PL, has developed a chatbot that makes an automated phone call to the final recipient to inform them of the exact time and point of delivery of their order. The chatbot automatically calls the recipient when the delivery driver begins the route to the final delivery point. The robot, which receives the notification, traces the route through Google Maps, calculates how long the carrier will take, and then makes the call informing the consumer of the exact arrival time at the agreed delivery point.
The system allows the customer to repeat the message left by the robot or cancel the delivery. In the latter case, the carrier receives notification that the customer will not be at home, preventing an unsuccessful delivery.
Using AI to create true personalisation
Creating true personalisation is one of the most potentially significant uses of AI in last-mile delivery, providing customers with the right delivery and returns options at the right price, throughout their experience. This could be delivered through a parcel carrier providing an online checkout tool, recommending the optimum delivery option based on previous delivery information. That would appeal to retailers, driving checkout conversion optimisation by tailoring one of the most important factors in ecommerce conversion (delivery) to each shopper.
Machine learning, as opposed to human-configured rules, allows a platform to consider potentially hundreds of data points when suggesting a recommended delivery option for a customer. This has the potential to be incredibly powerful and scalable for carriers and retailers, but requires accurate and structured data sources to provide a genuine alternative to a simple rules-based system.
The largest global logistic organisations already have access to the necessary data and the operational scale to make this a viable option. Amazon are constantly using data to improve their customer experience through product recommendations. If a customer puts an item in their cart or even just takes a look at it, Amazon will use that data. That way they can learn what each customer wants and likes and can recommend that same product or similar ones to them when they return to the shop. This is how they earn 35% of their annual sales. Customer personalisation and big data is no longer just a trend. It’s fundamental if you want to understand your consumers.
A realistic vision
In the coming years, AI is expected to play an increasingly prominent role in last-mile delivery tech, shaping a more efficient, customer-centric, and sustainable delivery ecosystem. However, the vagaries and challenges of B2C last-mile delivery will always require human input and ingenuity. That’s not to mention the power of friendly interaction and service with a smile, which greatly affects how customers experience their delivery. In practice, most successful last-mile automation won’t be focused on replacing the human factor, but on improving productivity, removing friction and reducing costs. As AI in last-mile tech evolves, its implementation will be a careful balance between innovation, practicality, and ethical considerations.