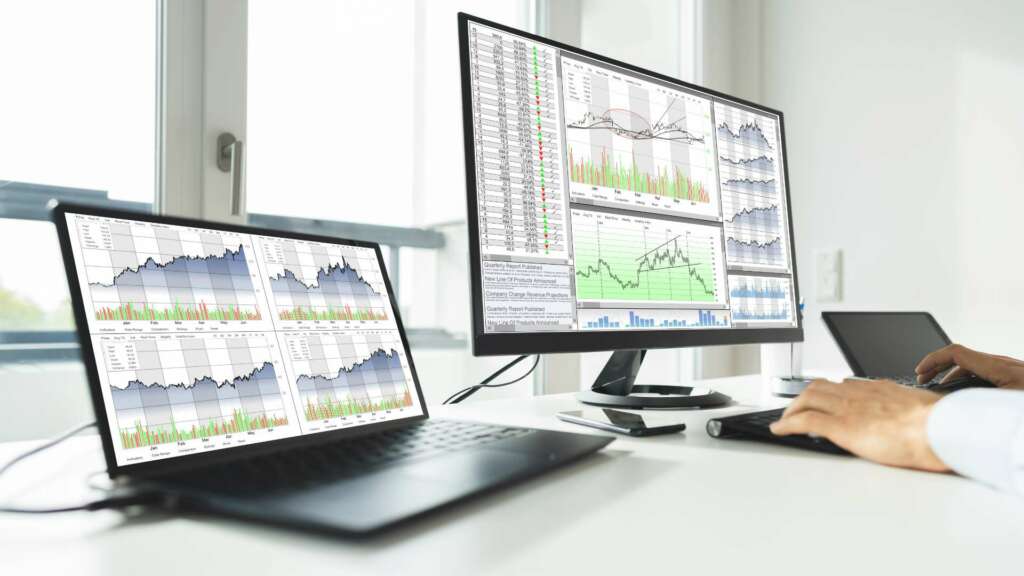
By Chris Sparrow, Head of Trade Analytics, Trading Solutions at FactSet
The world of a buy-side trader continues to evolve at a rapid pace. New skill sets are necessary for traders to process and analyze the many datasets that affect liquidity and trading outcomes. As the volume and breadth of data being presented to traders continues to grow, they are expected to efficiently process and filter the information that crosses their desk.
While data may be the new oil, if not properly handled, like oil, it can quickly create a mess. Traders are expected to use this data to show the value they add to implementing orders. Data is double edged- on one hand it can be used by the trader to improve implementation outcomes, but on the other hand data can be used against the trader to show missed opportunities.
This means that traders need both skills and tools to master data management. The data have valuable insights if properly mined using tools like SQL and python, so traders need to develop programming and analysis skills to put these tools to use. To deliver full value, traders need to be experts at not only sourcing liquidity, but also in the curation and interrogation of the data related to trade implementation. Armed with insights derived from their analysis combining their understanding of liquidity and the analysis of their trading performance, they can contribute to the overall investment process including facilitating discussions around implementation details with both portfolio managers and brokers.
The execution management system (EMS) is a key tool used by traders to execute their orders. The EMS has a nervous system of FIX connections linking the blotters in the EMS with a suite of destinations that the trader can choose to interact with.
But having a nerve center is not enough – the EMS also needs a long-term memory. The EMS should be able to understand the context of our trading. What we mean by context is to measure the liquidity environment and any other factors, such as order constraints, that play a role in performance. The EMS can remember the context by subscribing to analytics related to liquidity and making these historical liquidity analytics part of the pre-trade analysis and execution decision making.
This gets us closer to an analogy of a brain. An EMS with long-term memory can perform another key function that a brain performs; it can learn. By remembering past outcomes of trading in context with the prevailing liquidity in the market, the EMS can develop models that learn things like what market impact we can expect. In the EMS world, we do this by incorporating machine learning (ML) or deep learning (DL) techniques when analyzing our past outcomes.
As a platform with integrated access to past performance, the EMS becomes the central hub to quantify best execution. When deciding where, when, and how much to route, the trader can have access to an EMS that provides a data-driven approach that can incorporate constraints and conditions. A single system combining transaction cost analysis, liquidity analysis, and alternative data presented clearly to the trader empowers the trader to focus on their domain knowledge and less on integrating information from various sources.
The EMS is the best place for this type of integrated platform, because its nerve center is handing the order flow. Traders that have easy access to liquidity reports and model predictions along with access to past performance of similar orders or baskets can use that information to optimize their order flow. While the industry is abuzz with inter-op solutions, it is worth remembering that integration trumps inter-op.
In a fully integrated system, relevant analytics can be delivered to the EMS based on what is on the blotter and then visualized alongside to help traders decide how to route their flow. Examples include predicting spreads, volumes, and volatilities, observing trends, and how these liquidity factors vary together. Answering the question: how does the liquidity for the security (or basket) being traded compare with recent history? How does it compare with what we predicted? What strategies worked best in the past when liquidity conditions were like they are now?
The process of ML in the EMS is akin to the EMS developing a gut feel. Human traders, with their much more sophisticated reasoning power, develop a gut feel over time through experience. By trading many orders in many different conditions, traders learn what behaviors work best in various scenarios. As these skills develop, the traders can make quick decisions about how to handle situations that arise – they don’t need to think about it once they become experts. That means they can put more cognitive horsepower into other factors. It is only artificial (intelligence) to get the EMS to do something similar.
The EMS learns (via ML) and makes recommendations by combining historical analytics and presenting the results to the trader. The trader can continue to filter these recommendations, while over time, increased responsibility can be given to the EMS as the learning process continues and confidence is developed in the recommendations. In this way the EMS handles the task of gathering data and turning it into information, while the trader uses their domain knowledge to take informed actions.